AI Bias: Malice of The Machines and What Can We Do About It
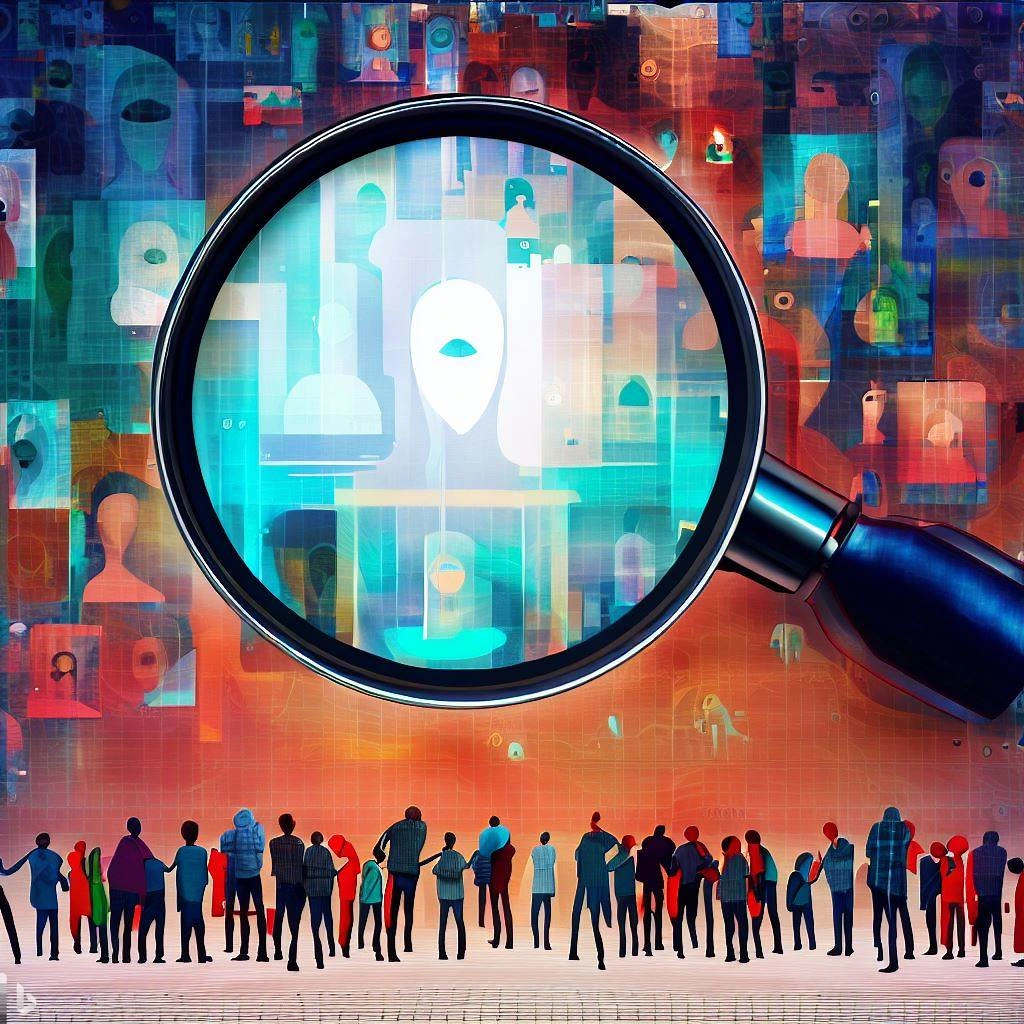
Northpointe—a private company formerly called Equivant created the Correctional Offender Management System(COMPAS)–a computer program that uses an algorithm to assess the risk of defendant reoffending.
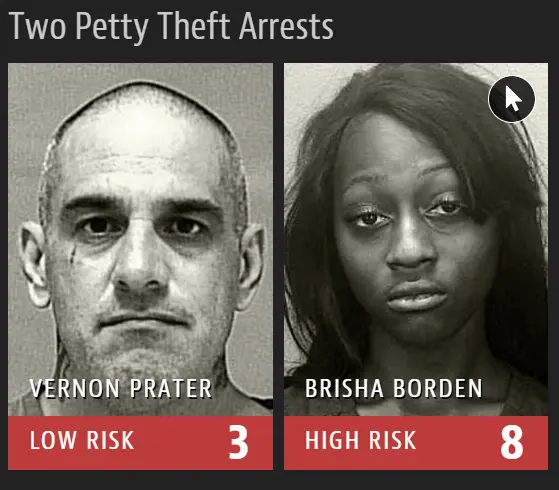
ProPublica analyzed COMPAS assessments for over 7,000 arrestees in Broward County, Florida, and discovered that black defendants were more than 2 times more likely to be labeled a criminal or at high risk than white defendants, but here’s the thing…
White defendants were actually at a higher risk and much more likely to end up with charges than the black ones pointed out, which procures that the AI is biased against black defendants.
And this is not just the first case, several chatbots like ChatGPT, Bard, Google, or Amazon’s selective preference has been proven time and time again as a core bias against a certain group.
AI bias is unfair and systematic discrimination of certain individuals or groups, intentionally or unintentionally.
The bias can creep into the algorithm and when AI systems learn to make decisions off the training data, that are inclusive of human decisions or reflect historical or social inequities, even sensitive remarks or variables such as gender, race, or sexual orientation are removed.
AI Bias is a Signal for Bigger Chaos…
AI algorithms are too ‘on the dot’ sometimes for example, you ask a cook to prepare a meal for you and say, “I’d like something delicious.” The cook responds, “Alright Delicious, what’s your next request?”.
You see algorithms much like the cook mentioned follow instructions quite literally and this can craft disparity between our outcome desired and the precise instructions. The difference itself is called label choice bias.
AI bias can propose multiple negative impacts on people’s lives, rights, and opportunities in various domains.
The dataset used to train these AI systems may perpetuate existing bias through previous discriminatory patterns and harm individuals or communities—be it giving higher risk scores to African Americans in the criminal justice system or categorizing them under the derogatory term ‘Gorillas’ in Google Photos.
It can deny loans to individuals from a minority caste or community and even limit their opportunities in the educational system by opting them out of admission enrollment.
Causes of AI bias
In 1988, AI bias was first recorded when a British medical school was found guilty of having a computer program that discriminated against women and non-European names who were applicants wanting to be invited for an interview round.
The program was biased and didn’t qualify the respective group to be interviewed. AI bias takes many forms:
- Data bias: It occurs when the AI is trained on limited data and is unable to serve the wider population it’s supposed to work for. For example AI system is trained on data that only includes men but it’s supposed to serve women as well. In that case, it may not perform well for the female group.
- Algorithmic bias: Algorithmic bias is when the algorithm in AI system is designed in a way that favors a certain group over another just as some AI systems favor men or white people or women and people with darker skin tones.
- Human bias: It’s a pretty eerie and phenomenal system that can have its own bias based on the bias of its user for example, if a hiring manager has a bias against certain people, the AI system can also discriminate against those groups.
- Societal bias: AI system that reflects the biases of society. Let’s say the elites are discriminatory against a certain caste then the AI system trained would also possess partial decisions against the caste.
AI bias can lead to greater consequences where the group being constantly discriminated against may walk out for protests or conduct massacres as a sign of rebellion.
Solutions to AI bias
Andrew Wycoff—the director of OECD’s Directorate for Science, technology, and Innovation, OECD’s digital economy policy division economist said.
“Twelve years from now, we will benefit from radically improved accuracy and efficiency of decisions and predictions across all sectors. Machine learning systems will actively support humans throughout their work and play. This support will be unseen but pervasive – like electricity.”
This shows that scientists are working and proposing ways to mitigate the risks of machine learning bias. Here are some strategies that may help procure a better AIxhuman interaction:
1. Data Quality and Diversity
We must ensure that the training data used to train AI systems is diverse and representative of the population by collecting data from multiple sources and verifying it for being balanced and unbiased.
The use of more diverse training data has been shown to improve the accuracy of facial recognition systems for people of color.
2. Algorithmic Transparency and Accountability
If we turn to create more transparent algorithms and decision-making processes rather than the usual black box fallacy, then we might be able to understand our users and reduce the bias our systems may have toward the people.
European Union Agency for Fundamental Rights recommends that organizations using AI conduct regular audits to identify and address potential biases.
3. Human Oversight and Involvement
It can supervise the decisions made by AI systems by involving humans in the development and employment of systems, such as through human-in-the-loop approaches or by having human experts review the decisions made by AI systems. This can be especially helpful for medical diagnosis to improve accuracy.
4. Ethical Principles and Standards
Machine learning enthusiasts can develop codes of conduct and ethical guidelines for AI developers and users.
The IEEE Global Initiative on Ethics of Autonomous and Intelligent Systems has developed a set of ethical principles for the development and deployment of AI systems.
All these strategies and methods can help mitigate the risk of AI bias to ensure data quality and diversity, while human oversight and involvement can provide a check on the decisions made by AI systems.
Final Thoughts
In 2014, Google provided users with four genders: “male”, “female”, “rather not to say” and “custom”. They also added the “rather not to say” option for the users who’re reluctant to reveal their identity and “custom” is meant for the nonconventional gender groups.
This is an exemplary step for creating an AI that ain’t biased and is actually designed for all age groups, caste systems, races, and gender.
The steps by European agencies and research centers to mitigate AI will reduce the chances of an arising problem and prevail in a better dystopian future…