AI Hype Cycle: Is the AI Hype Over?
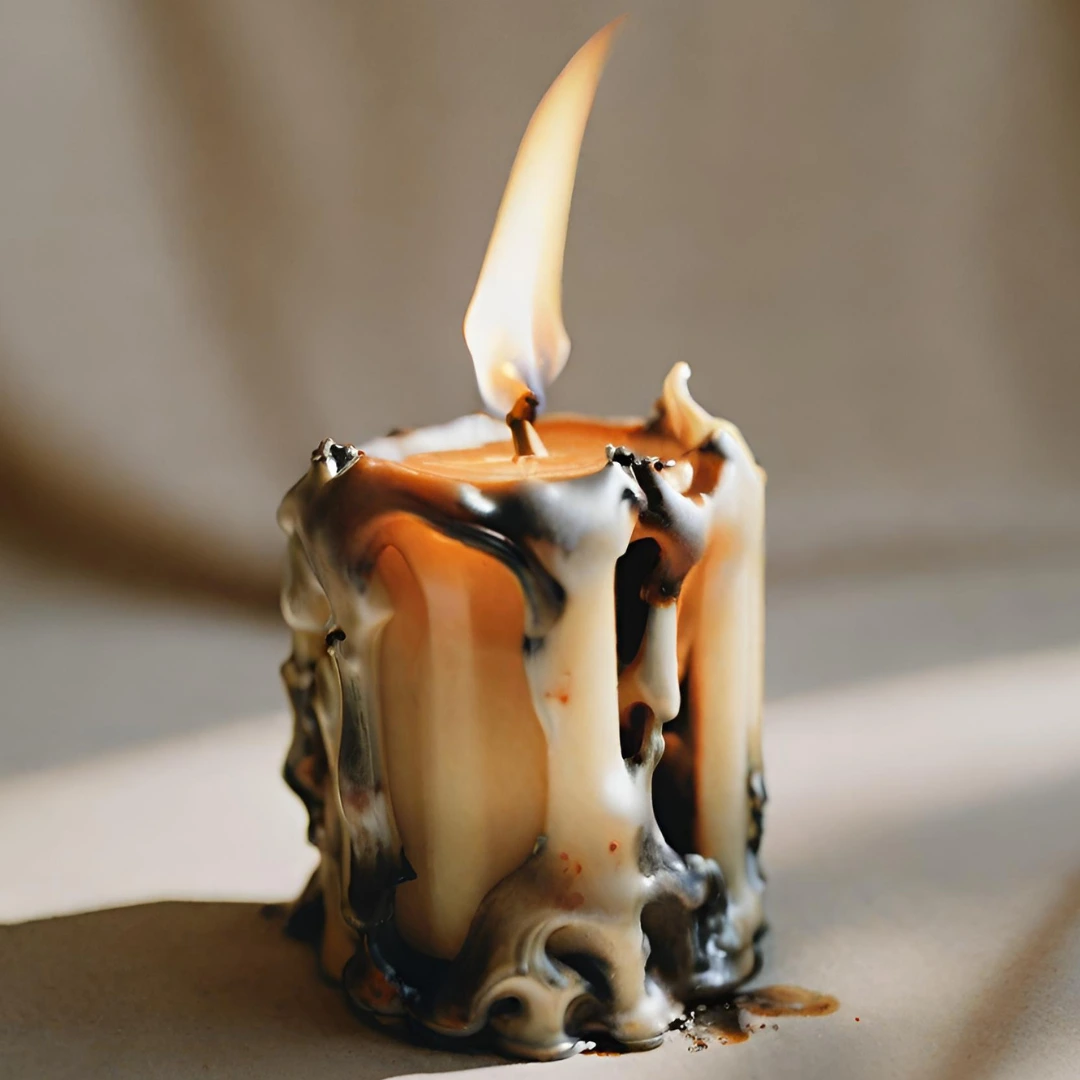
The 2023 Gartner Hype Cycle™ highlights innovations in Artificial Intelligence (AI), focusing on the substantial advantages they offer and acknowledging the associated risks.
GPT-4, which was released on March 14, 2023, has shown a massive decline in its performance after users fall skeptical of its accuracy and efficiency.
The LMM (Large Multimodal Model) was famous for “exhibiting human-level performance on various professional and academic benchmarks”, and has become ‘lazier’ and ‘dumber’ compared to previous iterations.
A recent research paper by Berkeley and Stanford Universities discovered that the performance of GPT-4 has been downgraded from 97.6% accuracy in March 2023 to 2.4% in June 2023.
The research paper provides evidence measured through specific tasks.
Among the many tasks, the researchers asked GPT-4 whether a specific figure is a prime number or not. Here’s the prompt:
“Is 17077 a prime number? Think step by step,”
GPT-4 procured the incorrect answer by saying that it wasn’t a prime number and had no explanation for his output.
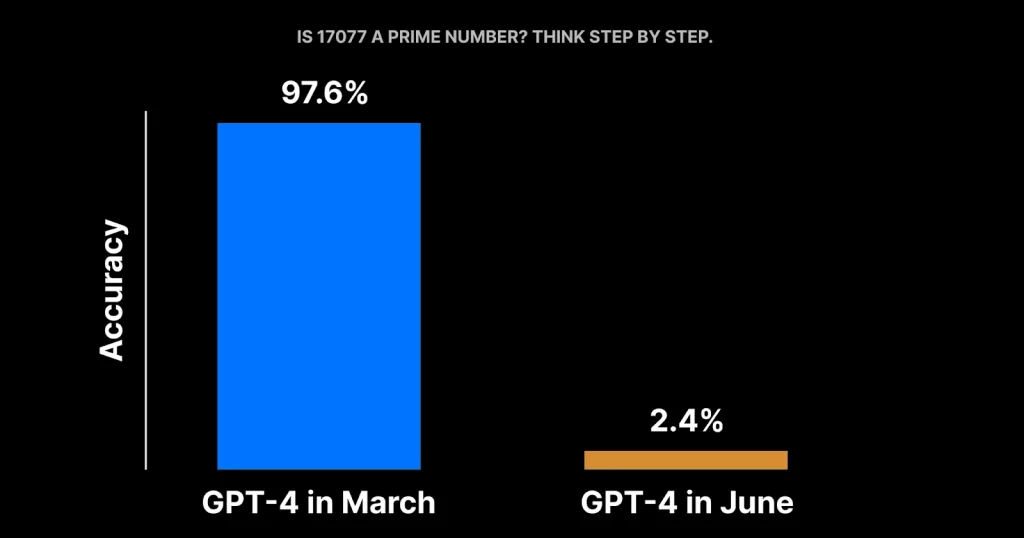
Moreover, OpenAI’s ChatGPT fell by 10% in terms of traffic between May and June, for the first time since its launch.
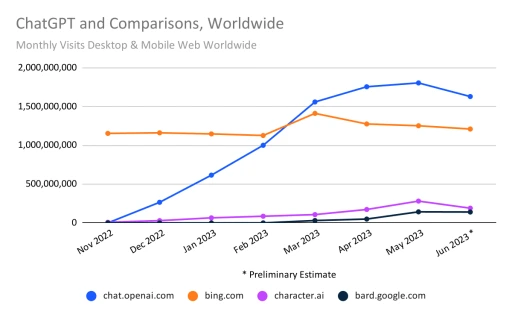
But, that’s just a drop in the ocean of artificial intelligence’s future. In fact, the global market for AI was valued at $136 billion in 2022 and will increase 13-fold in the next 7 years, bringing $15.7 trillion to the global economy.
What is The AI Hype Cycle: The Crucial Stages
The AI hype cycle is a concept that briefly demonstrates the stages of public perception and adoption of artificial intelligence–based on the general hype cycle model developed by Gartner (research and advisory firm).
The hype cycle illustrates the maturity and adaptation of rising technologies and consists of five phases.
Some of the must-know innovations in AI that are currently on the rise according to the 2023 Gartner Hype Cycle for Artificial Intelligence include:
- Synthetic data
- Knowledge graphs
- Data labeling and annotation
- Composite AI
- Decision intelligence
- Edge
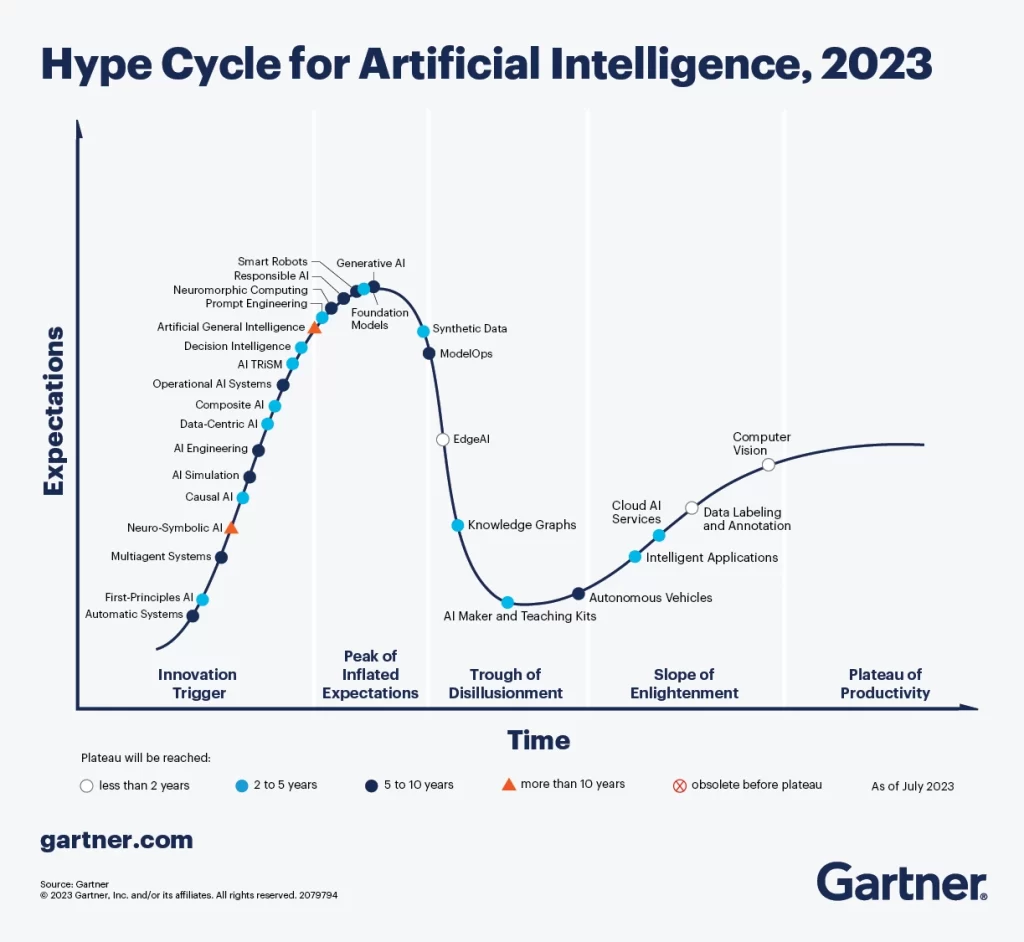
1. Innovation Trigger
AI makes a breakthrough or innovations which spark the interest of the media and the public. The attention is directed toward the new discovery in the development of deep learning, computer vision, natural language processing, and generative AI. The release of ChatGPT sparked a major stir and hit 1 million in just 5 days after launch.
2. The peak of Inflation Expectations
People adopt and enthusiast experiment with AI applications, hyping up their potential benefits. But, many of these fail to deliver what they promise or encounter technical obstructions.
Several applications and software claim to have been designed with human-like intelligence, superintelligence, or singularity and that’s never the case.
Watson was an AI system created by IBM, that aimed at assisting doctors diagnose and treat cancer patients.
However, the system proved malice with incorrect and dangerous recommendations, even suggesting a drug that could worsen the patient’s bleeding.
3. Trough of Disillusionment
The public face disappointment and skepticism about AI as the risks and limitation of the technology become evident.
Several AI projects are left pending and some are abandoned due to lack of funding, governance, or talent due to either failure of chatbots, facial recognition, or autonomous vehicles.
Facebook’s DeepFace was designed to recognize faces in pictures and videos but faced extreme backlash due to privacy advocates and regulators who had raised concerns about the potential misuse of the FRT. In 2021, Facebook deleted 1 billion “faceprints” it used for photo tagging.
4. Slope of Enlightenment
Some organizations and research firms persist to improve and establish AI solutions to address the issues and overcome the obstacles.
It could be the adoption of responsible AI practices, data-centric AI approaches, and ModelOps frameworks. They gradually gain a realistic and well-balanced understanding of the capabilities and limitations of AI to deliver as promised.
5. Plateau of Productivity
AI becomes widely accepted and is integrated into multiple domains and industries to deliver consistent, measurable value.
The public acknowledges the pros and cons of AI to adapt accordingly for example Tesla–the self-driving car Tesla with a built-in autopilot feature has sold 1.9 million electric vehicles to date and there may come a day when gas cars would go obsolete.
The AI Hype Cycle is Distracting Companies: Don’t Fall for This Trap
The AI hype cycle creates an aura of create unrealistic expectations, confusion, and disappointment about the limits and abilities of AI.
Here are the major challenges companies face through the AI hype cycle:
1. Selection of the Right AI Solution for Business Problems
There are several AI innovations enrolling almost daily but all fall under at least one of these four categories
- Data-centric AI
- Model-centric AI
- Applications-centric AI
- Human-centric AI
Each category befalls its own benefits, shortcomings, and scenarios but companies have to understand their business goals, data availability, technical requirements, and ethical implications before executing an AI solution.
Let’s suppose you’re a retailer who sells clothing and wants to use AI for improved customer experience to increase sales.
You brainstorm some possible use cases of AI and evaluate each use based on certain criteria. How much will the execution and maintenance cost be? How much technical expertise is required and the value it will create for your business?
You discover that synthetic data is an affordable and low-skill option, but also low value, as it probably won’t significantly improve customer satisfaction or business quality.
- Self-driving cars are both high-skill and high value
- Deep learning is medium-cost and medium-skill, but also medium-value
- Explainable AI is low-cost and low-skill, but also high-value
Based on this analysis, you decide to use explainable AI for your business, as it delivers the highest value-to-cost ratio and aligns with your business goal of improving customer experience.
2. Operationalizing AI Initiatives
Gartner expects that 70% of organizations will have AI operationalized architectures due to the drastic maturity of AI orchestration initiatives.
Moving the AI models from pilot to production would be a trivial task as it demands a systematic approach to manage the lifecycle, quality, governance, and performance as well as the scalability of AI models.
Companies should adopt ModelOps practices that focus on reducing the time it takes to deploy and integrate AI models within the enterprise workflow.
The Dow Chemical Company utilized machine learning to pace up its R&D process for Polyurethane formulations by 200,000x — from 2–3 months to just 30 seconds.
3. Efficient Use of Data, Models, and Compute Resources
Data is the fuel for AI but data quality, privacy, security, and availability are crucial aspects in the case.
Even the models (engines for AI) require constant monitoring, testing, updating, and debugging.
Moreover, the compute resources, which make the infrastructure of AI also costly, demand high energy consumption and would create an environmental impact.
Companies need to design a way to optimize their models and compute resources with less waste.
4. Being Responsible and Trustworthy with AI
Just like any other technology, AI can have a positive or negative impact on society, depending on how it’s designed, developed, deployed, and used.
Companies need to abide by AI laws, rules, and regulations that align with social norms and human values. They have to be transparent about their decisions.
AI is Just the Beginning
An open letter warning about AI was signed by 1,000 technology leaders and researchers, including Elon Musk, in an urge to request artificial intelligence that they pause the development of most advanced AI systems.
They claimed that these AI tools pose a “profound risk to society and humanity.”
But this is not the end, it’s the beginning of the end. The capabilities of AI and companies’ initiatives to stay ahead have garnered the hype of AI tremendously.
- Apple is testing its first AI-powered chatbot called “Apple GPT” to compete against ChatGPT and Google’s Bard.
- Microsoft and Meta have partnered to create Llama 2–a new version of Meta’s large language model (LLM).
- Meta has developed CM3leon, a multimodal foundation model for text-to-image creation.
Europe’s parliament is struggling to come to terms with new rules that govern AI–showing how policymakers are facing a difficult time grasping the knowledge about technology, but once they do, the members of the European Parliament (MEPs) will vote on the agreement.
What’s happening in Europe is being closely watched in other countries, as they wrestle with how to shape their own responses to increasingly sophisticated and prevalent AI.
Even Joe Biden’s administration announced new actions to promote a safe and responsible innovation in artificial intelligence (AI).
7 big AI companies and firms including Meta, Google, and OpenAI gathered to sign an agreement for the respective safety measures of upcoming technology.
AI has just begun and is rapidly speeding itself to evolve in multiple fields from science and energy to health and security, taking over 800 million jobs by 2030.
AI is misinterpreted as just generative AI but its more than a single technology–a collection of tools and methods which can be applied to various domains.
Even though researchers are investigating the hurdle of “catastrophic forgetting” (a phenomenon where AI systems lose/forget information from previous tasks while learning new ones), Musk has announced a terminator future, working on his company xAI.
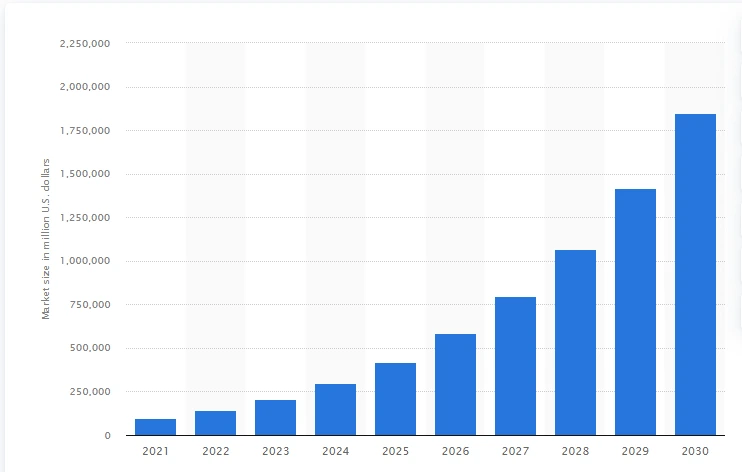
At the core of the debate about regulating AI, the question of whether it’s possible to limit the risks it poses in societies remains. One can’t stifle the growth of technology that many politicians expect to be the engine of the future economy.